ほとんどの AI モデルには少なくとも 1 つの共通点があります。それは、インターネット経由で転送されるリソースに対してかなり大規模であるということです。最小の MediaPipe オブジェクト検出モデル(SSD MobileNetV2 float16
)の重量は 5.6 MB、最大は約 25 MB です。
オープンソースの LLM gemma-2b-it-gpu-int4.bin
は 1.35 GB でクロックインします。これは LLM にとって非常に小さいものです。生成 AI モデルは巨大なものになり得ます。これこそが今日 AI がクラウドで
活用されている理由です高度に最適化されたモデルをデバイスで直接実行するアプリはますます増えています。ブラウザで実行されている LLM のデモはありますが、ブラウザで実行されている本番環境グレードの他のモデルの例を次に示します。
- Adobe Photoshop は、インテリジェントなオブジェクト選択ツールとして、デバイス上で
Conv2D
モデルのバリアントを実行します。 - Google Meet では、背景ぼかし機能のために人物セグメンテーション用に
MobileNetV3-small
モデルの最適化されたバージョンを実行しています。 - Tokopedia は、リアルタイムの顔検出に
MediaPipeFaceDetector-TFJS
モデルを実行して、サービスへの無効な登録を防ぎます。 - Google Colab では、Colab ノートブックでハードディスクのモデルを使用できます。
将来のアプリケーションの起動を高速化するには、暗黙的な HTTP ブラウザ キャッシュに依存するのではなく、デバイス上にモデルデータを明示的にキャッシュに保存する必要があります。
このガイドでは、gemma-2b-it-gpu-int4.bin model
を使用して chatbot を作成しますが、この方法は、デバイス上の他のモデルや他のユースケースに合わせて一般化できます。アプリをモデルに接続する最も一般的な方法は、他のアプリリソースとともにモデルを提供することです。配信の最適化は非常に重要です
適切なキャッシュ ヘッダーを構成する
サーバーから AI モデルを提供する場合は、正しい Cache-Control
ヘッダーを構成することが重要です。次の例は堅牢なデフォルト設定を示しており、アプリのニーズに合わせて構築できます。
Cache-Control: public, max-age=31536000, immutable
AI モデルの各リリース バージョンは静的リソースです。変更されないコンテンツには、リクエスト URL で長い max-age
とキャッシュ無効化を組み合わせて指定します。モデルを更新する必要がある場合は、新しい URL を指定する必要があります。
ユーザーがページを再読み込みすると、サーバーはコンテンツが安定していることを認識していても、クライアントは再検証リクエストを送信します。immutable
ディレクティブは、コンテンツが変更されないため再検証が不要であることを明示的に示しています。immutable
ディレクティブは、ブラウザ、中間キャッシュ、プロキシ サーバーで広くサポートされていませんが、広く普及している max-age
ディレクティブと組み合わせることで、最大限の互換性を確保できます。public
レスポンス ディレクティブは、レスポンスを共有キャッシュに保存できることを示します。
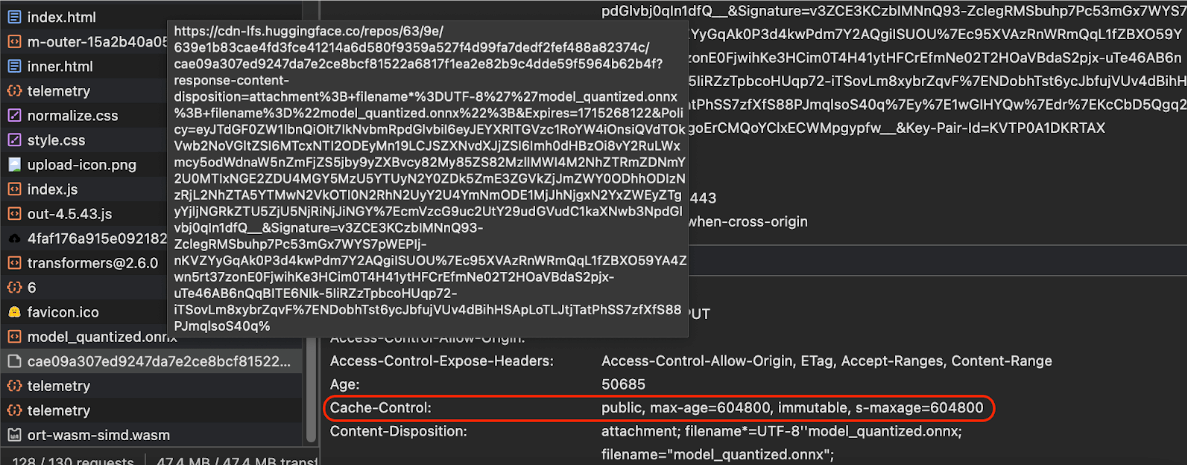
Cache-Control
ヘッダーが表示されます。(ソース)
AI モデルをクライアントサイドでキャッシュに保存する
AI モデルを提供する場合は、ブラウザ内にモデルを明示的にキャッシュに保存することが重要です。これにより、ユーザーがアプリを再読み込みした後にモデルデータを簡単に使用できるようになります。
これを達成するために使用できる手法はいくつかあります。次のコードサンプルでは、各モデルファイルがメモリ内の blob
という名前の Blob
オブジェクトに保存されていると仮定します。
パフォーマンスを理解するために、各コードサンプルには performance.mark()
メソッドと performance.measure()
メソッドのアノテーションが付けられています。これらの尺度はデバイスに依存するため、一般化することはできません。
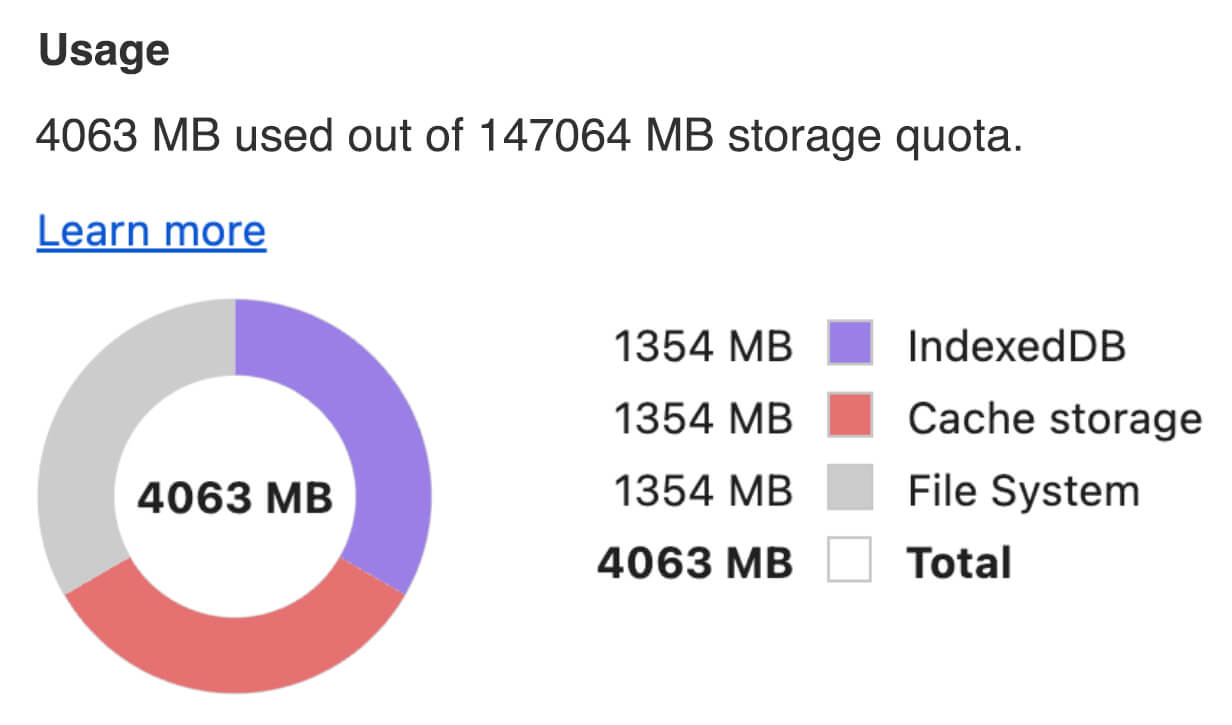
Cache API、Origin Private File System API、IndexedDB API のいずれかの API を使用して、ブラウザで AI モデルをキャッシュに保存することもできます。一般的には Cache API の使用が推奨されていますが、このガイドではすべてのオプションのメリットとデメリットについて説明します。
キャッシュ API
Cache API は、有効期間が長いメモリにキャッシュに保存される Request
オブジェクトと Response
オブジェクトのペアに永続ストレージを提供します。この API は Service Worker の仕様で定義されていますが、メインスレッドまたは通常のワーカーから使用できます。Service Worker コンテキスト外で使用するには、Request
オブジェクトではなく合成 URL と合成された Response
オブジェクトを組み合わせて、Cache.put()
メソッドを呼び出します。
このガイドでは、メモリ内 blob
を前提としています。キャッシュキーとして架空の URL を使用し、blob
に基づく合成 Response
を使用します。モデルを直接ダウンロードする場合は、fetch()
リクエストを行って取得した Response
を使用します。
たとえば、Cache API を使用してモデルファイルを保存および復元する方法を次に示します。
const storeFileInSWCache = async (blob) => {
try {
performance.mark('start-sw-cache-cache');
const modelCache = await caches.open('models');
await modelCache.put('model.bin', new Response(blob));
performance.mark('end-sw-cache-cache');
const mark = performance.measure(
'sw-cache-cache',
'start-sw-cache-cache',
'end-sw-cache-cache'
);
console.log('Model file cached in sw-cache.', mark.name, mark.duration.toFixed(2));
} catch (err) {
console.error(err.name, err.message);
}
};
const restoreFileFromSWCache = async () => {
try {
performance.mark('start-sw-cache-restore');
const modelCache = await caches.open('models');
const response = await modelCache.match('model.bin');
if (!response) {
throw new Error(`File model.bin not found in sw-cache.`);
}
const file = await response.blob();
performance.mark('end-sw-cache-restore');
const mark = performance.measure(
'sw-cache-restore',
'start-sw-cache-restore',
'end-sw-cache-restore'
);
console.log(mark.name, mark.duration.toFixed(2));
console.log('Cached model file found in sw-cache.');
return file;
} catch (err) {
throw err;
}
};
送信元の Private File System API
オリジン プライベート ファイル システム(OPFS)は、ストレージ エンドポイントの比較的新しい標準です。通常のファイル システムとは異なり、公開元が非公開であるため、ユーザーに表示されません。パフォーマンスを重視して高度に最適化された特別なファイルにアクセスし、そのコンテンツへの書き込みアクセス権を付与します。
たとえば、OPFS でモデルファイルを保存して復元する方法を次に示します。
const storeFileInOPFS = async (blob) => {
try {
performance.mark('start-opfs-cache');
const root = await navigator.storage.getDirectory();
const handle = await root.getFileHandle('model.bin', { create: true });
const writable = await handle.createWritable();
await blob.stream().pipeTo(writable);
performance.mark('end-opfs-cache');
const mark = performance.measure(
'opfs-cache',
'start-opfs-cache',
'end-opfs-cache'
);
console.log('Model file cached in OPFS.', mark.name, mark.duration.toFixed(2));
} catch (err) {
console.error(err.name, err.message);
}
};
const restoreFileFromOPFS = async () => {
try {
performance.mark('start-opfs-restore');
const root = await navigator.storage.getDirectory();
const handle = await root.getFileHandle('model.bin');
const file = await handle.getFile();
performance.mark('end-opfs-restore');
const mark = performance.measure(
'opfs-restore',
'start-opfs-restore',
'end-opfs-restore'
);
console.log('Cached model file found in OPFS.', mark.name, mark.duration.toFixed(2));
return file;
} catch (err) {
throw err;
}
};
IndexedDB API
IndexedDB は、ブラウザに任意のデータを永続的に保存するための確立された標準です。IndexedDB はやや複雑な API で知られていますが、idb-keyval などのラッパー ライブラリを使用することで、IndexedDB を従来の Key-Value ストアのように扱うことができます。
次に例を示します。
import { get, set } from 'https://cdn.jsdelivr.net/npm/idb-keyval@latest/+esm';
const storeFileInIDB = async (blob) => {
try {
performance.mark('start-idb-cache');
await set('model.bin', blob);
performance.mark('end-idb-cache');
const mark = performance.measure(
'idb-cache',
'start-idb-cache',
'end-idb-cache'
);
console.log('Model file cached in IDB.', mark.name, mark.duration.toFixed(2));
} catch (err) {
console.error(err.name, err.message);
}
};
const restoreFileFromIDB = async () => {
try {
performance.mark('start-idb-restore');
const file = await get('model.bin');
if (!file) {
throw new Error('File model.bin not found in IDB.');
}
performance.mark('end-idb-restore');
const mark = performance.measure(
'idb-restore',
'start-idb-restore',
'end-idb-restore'
);
console.log('Cached model file found in IDB.', mark.name, mark.duration.toFixed(2));
return file;
} catch (err) {
throw err;
}
};
ストレージを永続としてマーク
これらのキャッシュ メソッドの最後に navigator.storage.persist()
を呼び出して、永続ストレージを使用する権限をリクエストします。このメソッドは、権限が付与されている場合は true
に解決され、そうでない場合は false
に解決される Promise を返します。ブラウザは、ブラウザ固有のルールに応じて、リクエストを尊重する場合と尊重しない場合があります。
if ('storage' in navigator && 'persist' in navigator.storage) {
try {
const persistent = await navigator.storage.persist();
if (persistent) {
console.log("Storage will not be cleared except by explicit user action.");
return;
}
console.log("Storage may be cleared under storage pressure.");
} catch (err) {
console.error(err.name, err.message);
}
}
特殊なケース: ハードディスク上のモデルを使用する
ブラウザのストレージの代わりに、ユーザーのハードディスクから AI モデルを直接参照できます。この手法は、研究に重点を置いたアプリが特定のモデルをブラウザで実行する可能性を示すのに役立ち、アーティストは専門的なクリエイティビティ アプリで自己トレーニングされたモデルを使用できます。
File System Access API
File System Access API を使用すると、ハードディスクからファイルを開き、IndexedDB に保持できる FileSystemFileHandle を取得できます。
このパターンでは、ユーザーはモデルファイルへのアクセスを 1 回付与するだけで済みます。永続的な権限により、ユーザーはファイルへのアクセスを永続的に許可することを選択できます。アプリを再読み込みし、マウスクリックなどの必要なユーザー操作を行うと、ハードディスク上のファイルにアクセスして IndexedDB から FileSystemFileHandle
を復元できます。
必要に応じてファイル アクセス権限がクエリされ、リクエストされます。これにより、今後の再読み込みがシームレスになります。次の例は、ハードディスクからファイルのハンドルを取得し、そのハンドルを保存して復元する方法を示しています。
import { fileOpen } from 'https://cdn.jsdelivr.net/npm/browser-fs-access@latest/dist/index.modern.js';
import { get, set } from 'https://cdn.jsdelivr.net/npm/idb-keyval@latest/+esm';
button.addEventListener('click', async () => {
try {
const file = await fileOpen({
extensions: ['.bin'],
mimeTypes: ['application/octet-stream'],
description: 'AI model files',
});
if (file.handle) {
// It's an asynchronous method, but no need to await it.
storeFileHandleInIDB(file.handle);
}
return file;
} catch (err) {
if (err.name !== 'AbortError') {
console.error(err.name, err.message);
}
}
});
const storeFileHandleInIDB = async (handle) => {
try {
performance.mark('start-file-handle-cache');
await set('model.bin.handle', handle);
performance.mark('end-file-handle-cache');
const mark = performance.measure(
'file-handle-cache',
'start-file-handle-cache',
'end-file-handle-cache'
);
console.log('Model file handle cached in IDB.', mark.name, mark.duration.toFixed(2));
} catch (err) {
console.error(err.name, err.message);
}
};
const restoreFileFromFileHandle = async () => {
try {
performance.mark('start-file-handle-restore');
const handle = await get('model.bin.handle');
if (!handle) {
throw new Error('File handle model.bin.handle not found in IDB.');
}
if ((await handle.queryPermission()) !== 'granted') {
const decision = await handle.requestPermission();
if (decision === 'denied' || decision === 'prompt') {
throw new Error(Access to file model.bin.handle not granted.');
}
}
const file = await handle.getFile();
performance.mark('end-file-handle-restore');
const mark = performance.measure(
'file-handle-restore',
'start-file-handle-restore',
'end-file-handle-restore'
);
console.log('Cached model file handle found in IDB.', mark.name, mark.duration.toFixed(2));
return file;
} catch (err) {
throw err;
}
};
これらの方法は相互に排他的ではありません。ブラウザにモデルを明示的にキャッシュし、ユーザーのハードディスクにあるモデルを使用する場合があります。
デモ
MediaPipe LLM デモでは、3 つの通常のケース ストレージ メソッドとハードディスク メソッドがすべて実装されています。
参考: 大きなファイルをチャンク形式でダウンロードする
インターネットから大規模な AI モデルをダウンロードする必要がある場合は、ダウンロードしたデータを別々のチャンクに並列化してから、クライアント上で再度つなぎ合わせます。
コードで使用できるヘルパー関数を以下に示します。url
を渡すだけで済みます。chunkSize
(デフォルト: 5 MB)、maxParallelRequests
(デフォルト: 6)、progressCallback
関数(downloadedBytes
と合計 fileSize
をレポート)、AbortSignal
シグナルの signal
はすべて省略可能です。
プロジェクトに次の関数をコピーするか、npm パッケージから fetch-in-chunks
パッケージをインストールします。
async function fetchInChunks(
url,
chunkSize = 5 * 1024 * 1024,
maxParallelRequests = 6,
progressCallback = null,
signal = null
) {
// Helper function to get the size of the remote file using a HEAD request
async function getFileSize(url, signal) {
const response = await fetch(url, { method: 'HEAD', signal });
if (!response.ok) {
throw new Error('Failed to fetch the file size');
}
const contentLength = response.headers.get('content-length');
if (!contentLength) {
throw new Error('Content-Length header is missing');
}
return parseInt(contentLength, 10);
}
// Helper function to fetch a chunk of the file
async function fetchChunk(url, start, end, signal) {
const response = await fetch(url, {
headers: { Range: `bytes=${start}-${end}` },
signal,
});
if (!response.ok && response.status !== 206) {
throw new Error('Failed to fetch chunk');
}
return await response.arrayBuffer();
}
// Helper function to download chunks with parallelism
async function downloadChunks(
url,
fileSize,
chunkSize,
maxParallelRequests,
progressCallback,
signal
) {
let chunks = [];
let queue = [];
let start = 0;
let downloadedBytes = 0;
// Function to process the queue
async function processQueue() {
while (start < fileSize) {
if (queue.length < maxParallelRequests) {
let end = Math.min(start + chunkSize - 1, fileSize - 1);
let promise = fetchChunk(url, start, end, signal)
.then((chunk) => {
chunks.push({ start, chunk });
downloadedBytes += chunk.byteLength;
// Update progress if callback is provided
if (progressCallback) {
progressCallback(downloadedBytes, fileSize);
}
// Remove this promise from the queue when it resolves
queue = queue.filter((p) => p !== promise);
})
.catch((err) => {
throw err;
});
queue.push(promise);
start += chunkSize;
}
// Wait for at least one promise to resolve before continuing
if (queue.length >= maxParallelRequests) {
await Promise.race(queue);
}
}
// Wait for all remaining promises to resolve
await Promise.all(queue);
}
await processQueue();
return chunks.sort((a, b) => a.start - b.start).map((chunk) => chunk.chunk);
}
// Get the file size
const fileSize = await getFileSize(url, signal);
// Download the file in chunks
const chunks = await downloadChunks(
url,
fileSize,
chunkSize,
maxParallelRequests,
progressCallback,
signal
);
// Stitch the chunks together
const blob = new Blob(chunks);
return blob;
}
export default fetchInChunks;
最適な方法を選択する
このガイドでは、ブラウザに AI モデルを効果的にキャッシュするさまざまな方法を見てきました。これは、アプリのユーザー エクスペリエンスとアプリのパフォーマンスを向上させるために重要なタスクです。Chrome ストレージ チームは、AI モデルにすばやくアクセスして読み込み時間を短縮し、応答性を向上させるため、最適なパフォーマンスを実現するために Cache API を推奨しています。
OPFS と IndexedDB はあまり使いにくいオプションです。OPFS API と IndexedDB API は、保存する前にデータをシリアル化する必要があります。また、IndexedDB はデータの取得時にデータのシリアル化を解除する必要があるため、大規模なモデルを保存するのに最適です。
ニッチなアプリケーションの場合、File System Access API を使用すると、ユーザーのデバイス上のファイルに直接アクセスでき、独自の AI モデルを管理するユーザーに最適です。
AI モデルを保護する必要がある場合は、サーバー上に保持します。クライアントに格納したら、DevTools または OFPS DevTools 拡張機能を使用して、Cache と IndexedDB の両方からデータを抽出するのは簡単です。これらのストレージ API のセキュリティは本質的に同じです。暗号化されたバージョンのモデルを保存しようとしても、クライアントに復号鍵を取得する必要がありますが、これはインターセプトされる可能性があります。つまり、不正な行為者がモデルを盗もうとする試みは、若干困難ですが、不可能ではありません。
アプリの要件、ターゲット ユーザーの行動、使用する AI モデルの特性に合わせてキャッシュ戦略を選択することをおすすめします。これにより、さまざまなネットワーク条件とシステムの制約に対して、アプリケーションの応答性と堅牢性を確保できます。
謝辞
このレビューは、Joshua Bell、Reilly Grant、Evan Stade、Nathan Memmott、Austin Sullivan、Etienne Noël、André Bandarra、Alexandra Klepper、François Beaufort、Paul Kinlan、Rachel Andrew がレビューしました。